We were really inspired by Google's drawing machine learning, and the ability to play around with the different types of applications that machine learning has in with drawing. In order to most quickly and accurately iterate over and over again, we started our explorations by playing around with the whiteboard. We started off playing around with the program to see if machine learning was able to detect the difference between written text and drawings. From this, we were also thinking of maybe incorporating mathematical graphs and/or equations as a possible third scope; an example that lives between text and drawing.
From our experiments, we saw that computer could usually detect between drawings and text, presumptuously mostly dependent on the text. The diversity of drawings was differed widely, as we literally began to draw just about everything that first came to mind, whereas text was definitely more limited in terms of aesthetic, and was visually more uniform. However, we came upon an interesting discovery when drawing stars, but in a linear form. Despite being drawings, it was detected as text, because of its linear nature. This propelled us into thinking about the possible implications for using machine learning to detect the differences between languages.
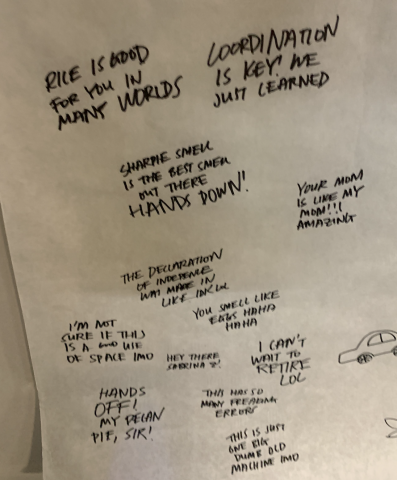
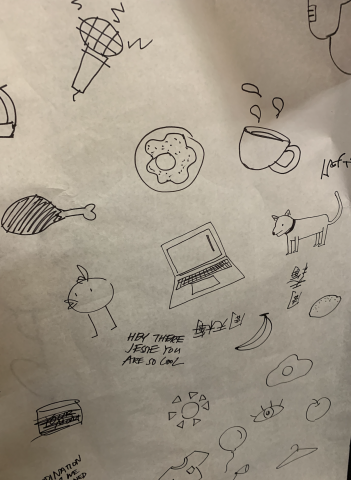
The stars that sparked off our stars.
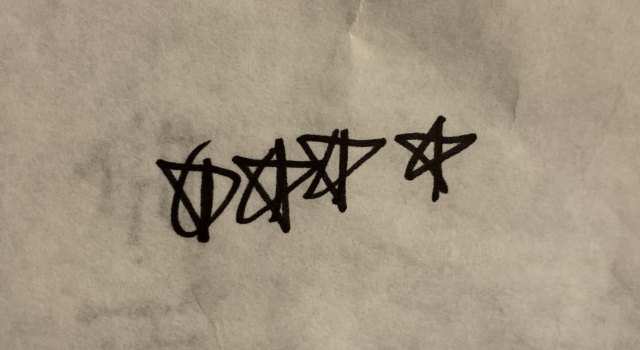
Our final exploration dealt with exploring the detecting the difference between western and eastern languages; western being more letter-based, and eastern being more pictorial-based characters.
Western Languages
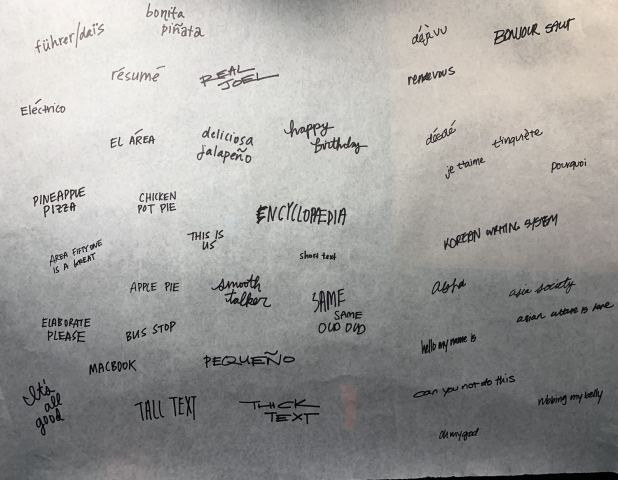
Eastern Languages

Training our model with white background, western text, and eastern text.
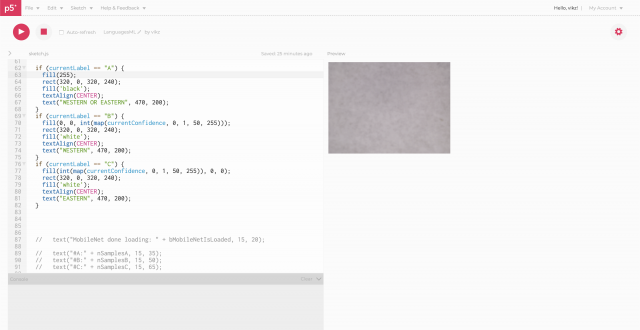
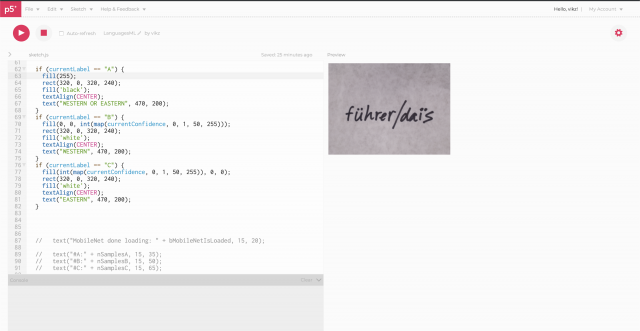

We decided to map the result out visually through three colors:
- White indicates that there is no hand written text being shown. (We fed a series of white background images to train this part)
- Blue indicates that it is more-so western text. (We fed a series of handwritten phrases and words in English, Spanish, French, and Latin to train this part)
- Red indicates that is more-so eastern text. (We fed a series of handwritten phrases and words in Chinese, Japanese, and Korean to train this part)
From our results, we've discovered a couple things.
The program is relatively good at detecting a "blank background" though a couple times, when our paper was shifted, the program recognized it as "western".
But most importantly, the program was very accurate in detecting western text, but significantly less so with eastern text.
This observation has led us to a couple hypotheses:
- Our data is lacking. We took about 100 photos for western and eastern each, but this may have been not enough for the machine learning to generative a conclusive enough database.
- The photos that we took could also have been of not high enough quality.
- In our sample data, we only wrote horizontally for western text, where as eastern had both horizontal and vertical.
Future thoughts...
To test the machine learning program to see if could simply tell the difference between western and eastern languages, we could do away with the "varied handwriting" completely and use very strict criteria (for handwriting style) in writing our sample text. When we tested the learned program, we could continue to write in that same style between the eastern and western texts. This could help isolate our variables to test out our above hypothesis.